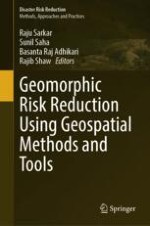
2024 | OriginalPaper | Buchkapitel
1. Landslide Susceptibility Assessment Based on Machine Learning Techniques
verfasst von : Jierui Li, Wen He, Lingke Qiu, Wen Zeng, Baofeng Di
Erschienen in: Geomorphic Risk Reduction Using Geospatial Methods and Tools
Verlag: Springer Nature Singapore
Aktivieren Sie unsere intelligente Suche, um passende Fachinhalte oder Patente zu finden.
Wählen Sie Textabschnitte aus um mit Künstlicher Intelligenz passenden Patente zu finden. powered by
Markieren Sie Textabschnitte, um KI-gestützt weitere passende Inhalte zu finden. powered by